1. Introduction
Agriculture is crucial to economic growth particularly in developing countries[1]. A 2022 report from the Food and Agriculture Organization of the United Nations (FAO) found that between 702 and 828 million people in the world faced hunger, and furthermore around 2.31 billion people, nearly one in three people in the world, were moderately or severely food insecure. Crop yields being negatively impacted by the occurrences of pests and diseases put extra pressure on increasing demands for agricultural productivity. In fact, plant pests and diseases that interfere with crop growth cause substantial losses, and destroy our food supply and agricultural industries[2, 3]. To mitigate the impacts, the monitoring of pests and diseases has been essential for taking proper prevention steps and optimizing control strategies. Besides, monitoring data is crucial to build prediction models to forecast potential outbreaks, and can accordingly guide decision-making[4]. Thus, the monitoring of plant pests and diseases in the early stages plays important roles to prevent crop loss and ensure food security.
Conventional monitoring of crop pests and diseases depends on visual inspection through field observation, with demands for skilled people and on-site visits, resulting in the labor intensive and low cost-effective systems and showing difficulties to detect pests and pathogens at the early onset of the symptom. Moreover, the measurements may not allow analyses on the spatial-temporal variability of pest damage and disease developments due to poor resolution and low efficiency. Molecular identification methods, such as polymerase chain reaction (PCR), are destructive in the sampling and diagnosis procedures, with the requirements of sample preparation, trained operators and expensive reagents. As agriculture is in the middle of the digital revolution[5], recent developments in agricultural technology have brought increasing demands for automated and non-destructive monitoring methods, which are expected to detect the pests and diseases at the early stage[6].
In the context of precision agriculture (PA), it is crucial to optimize resource usage for enhancing agricultural yields and minimizing hazards to environmental and human health[7-9]. Integration of innovative technologies can provide agriculture monitoring with accurate, high-resolution, multidimensional data to afford reliable prediction and early detection of pests and pathogens, eventually achieving sustainable precision crop protection. In detail, reliable data on the stress level of crops, presence of organisms, and identification of pests and diseases are needed to plan targeted plant protection strategies, thus preventing the spread of pests and diseases in a time manner. It is likely to obtain initial preliminary diagnoses through sensing technology for its capacity to detect plant stresses[10]. The array-based biosensors enable the transduction of biotic and abiotic variables such as plant physiology, metabolites, or microclimate into electrical signals for real-time monitoring. It is worth noted that sensing information needs to be linked directly to biological traits for more extensive application in the monitoring of crop pests and diseases[11, 12]. Furthermore, high-resolution data from biosensors would be used to develop prediction models and allow fine-scale insights of dynamics regarding morphology, behavior and physiology, providing baseline data for surveillance and implementation of control measures. In particular, pests damage and diseases developments are highly relevant to plant traits and environmental factors[13]. For instance, crop-environmental parameters, in combination with pests and pathogens dynamics, can be further processed to examine features of crucial events in the life cycle of those damaging organisms for occurrences and outbreaks forecasting[14]. It is crucial to investigate a multitude of biosensors for agriculture monitoring to enable pests or pathogens being detected early, in order to guide site-specific management and eradication programs.
Advanced biosensing technologies that could non-invasively, timely and automatically collect multidimensional data demonstrate a great potential to support biosecurity and agriculture surveillance[15]. Here we present an overview of state-of-the-art biosensing methods that can detect pests or diseases of agriculture crops, illustrating sensing mechanisms and representative applications, and also discuss future perspectives for agriculture monitoring, and the detection and identification of pests and pathogens in the crop production.
2. Emerging biosensing methodologies
2.1 Imaging-based technology
Since traditional survey methods have been labor-intensive, monitoring activities would face to a series of tradeoffs, such as reducing the number of locations and the frequency of sampling, leading to limited monitoring resolutions both spatially and temporally. Emerging technologies like automated image recognition methods offer potential solutions to revolutionize monitoring for more extensive coverage, thus provide more insights into population dynamics, distributions and interactions of insect pests and diseases[16]. Imaging-based techniques have been studied to detect and monitor plant stresses including pest infestation and disease development for decades. Advances in deep learning algorithms allow improvements of the automatic detection of pests and diseases on the basis of computer vision in order to reach higher efficiency and accuracy. A variety of symptomatic and asymptomatic changes would occur on plants in responses to pests and diseases. It is of paramount importance to early detect subtle or even invisible signs to minimize the negative effects. Various active and passive electro-optical sensors enable the detection of early changes in plant physiology. Imaging-based technologies, such as RGB/visible imaging, multi-spectral and hyperspectral sensors, thermal and fluorescence sensors, have provided a non-invasive and automated monitoring systems for detection and identification of insect pests and plant diseases[17, 18].
Healthy plants provide a higher reflection in the near infrared region and reflect less in the visible region, while infected plants increase their reflectance in the visible range and decrease slowly in the near infrared range. The biotic stress typically results in the variation of the reflectance that is possibly caused by a chlorophyll level reduction and transformations in internal structure[19]. Such changes may also occur before observable signs, leading to a timely measure. Infected plants show morphological and internal modifications that is highly specific, which enables detection and identification based on the spectral signature of plants. Notably, hyperspectral cameras can measure dozens to hundreds of narrow spectral bands across the electromagnetic spectrum to obtain images comprising of two spatial and one wavelength dimensions, therefore provide rich spectral information for detection, classification and quantification of diseases at an early age[20]. Marín-Ortiz et al.[21] identified the spectral variation (relevant spectral wavelengths) in tomato plants infected with Fusarium oxysporum, enabling detection during the incubation period in which the symptoms are not visible. Reflectance spectroscopy combined with linear discriminant models were able to discriminate infected plants from healthy ones with high accuracy (85%–93%), according to the changes in the reflectance of diseased leaves in the infrared range measured. Additionally, hyperspectral imaging was performed to detect maize plants that subjected to insect infestations, basing on the detectable changes in certain spectral bands of leaf reflectance profiles[22].
The utilization of smart sensor traps to monitor insect pests has been well-applied, such as acoustic detection for stored pests[23], or counting insect entry as the light is interrupted[24]. Nevertheless, imaging the trapped insects, often in combination with sticky trap or pheromone trap, is dominating in the development of automatic pest monitoring devices. Camera-based sensors use digital cameras for remote visual inspection of trapped insects for the real-time monitoring[25], or integrated with artificial intelligence techniques for automatic detection and counting of insect pests[26]. Pest detection systems capture the morphology, color, and texture through image processing. Such methods need a large number of annotated images that classified by expert entomologists to enhance image-identification algorithms, the accuracy needs further testing in the monitoring programs. Using camera to monitor insect pests, the image quality (e.g., resolution, illumination) should be ensured to assess insect presence and classification, particularly according to insect size and morphometric characteristics[4]. Besides, taking into accounts the energy supply and costs is necessary for the deployment of monitoring systems in the field. Shaked et al.[25] developed the semi-automatic trap with a 5-Mpixel camera (Omni Vision OV5647 NOIR Rasp Pi) to capture real-time images of the sticky plate surfaces that placed in the orchards, the entomologist classified the fruit flies based on the images with high-rate accuracy. Camera-based insect monitoring, paired with wireless sensor networks (WSNs), can greatly improve the probability of detection in the early stage of an incursion, and additionally allow predictions of outbreaks risk as well as report on the level of the pest population. In addition to image sensors, the optical sensors have proven to be effective in detecting insects via recording the wingbeat frequency based on disruption of the electric signal patterns caused by the partial occlusion of light from the wings, further facilitating insect identification through biometrics[27]. Potamitis et al.[28] presented a novel bimodal optoelectronic sensor based on Fresnel lenses and a stereo recorder, identifying the incoming insects from the optoacoustic spectrum profile of the wingbeat (Fig. 1).
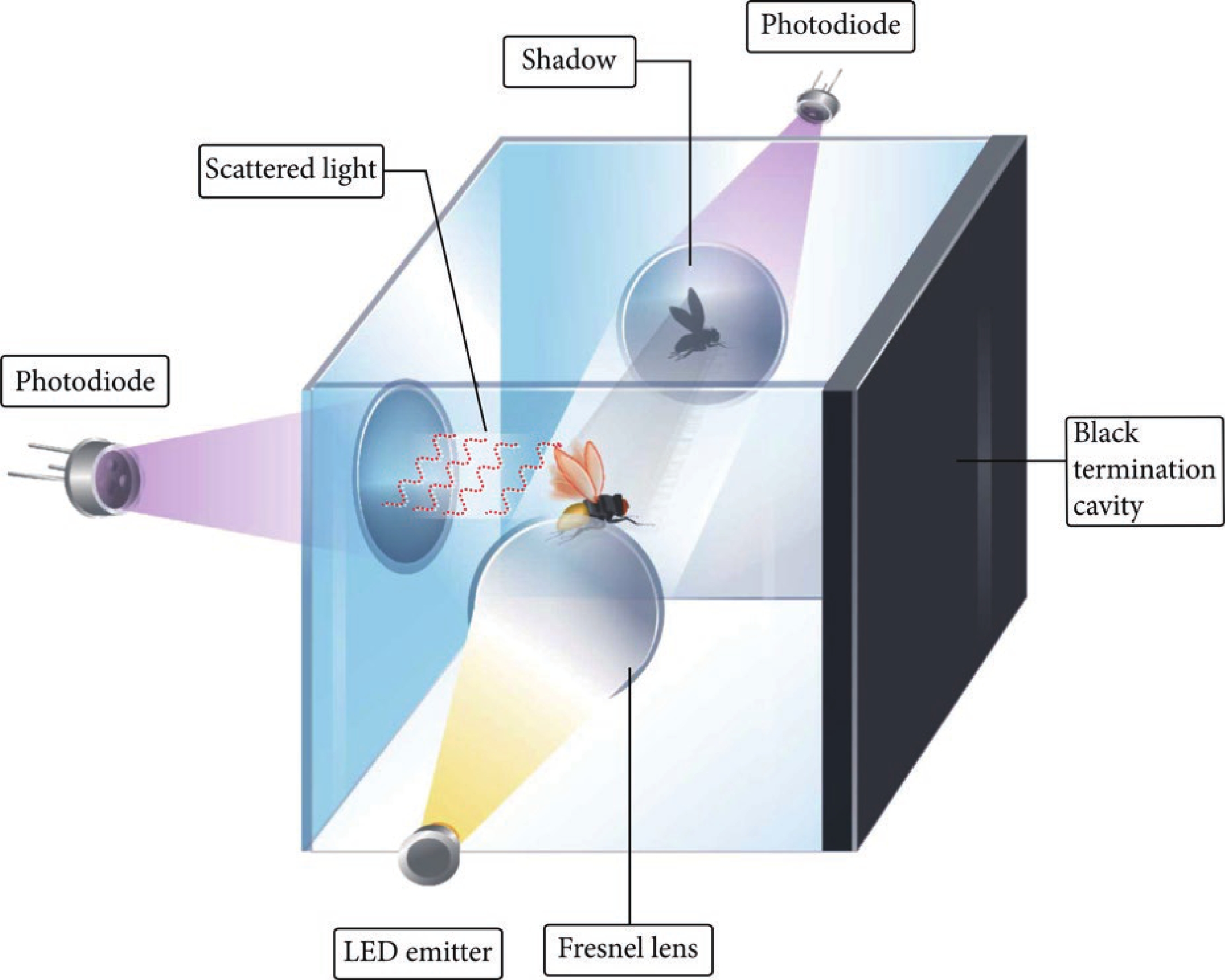
2.2 Electronic nose technology
It is desirable to capture physiological changes guided by interactions of pests and their hosts for early detection, because the variations may occur at very early stage of diseases. Volatile organic compounds (VOCs) are released from certain parts of plants into the atmosphere or the soil, mediating the communications between the plants and the surroundings. Plants produce a wide array of VOCs, that are one of the immediate responses of plants to pest infestation or pathogen infection, acting as a defense against pests by means of various mechanisms, such as reducing pest attacks or attracting predators[29]. The electronic nose (e-nose), mainly composed by an array of sensors, has been used to detect and discriminate volatile compounds for diseases detection. The rationale for e-nose is to detect the variation of VOC compositions when crops have been attacked. E-noses have already been applied for early detection of stored grain insects[30, 31] or storage diseases[32], fungus[33], bacterial diseases[34] and viruses[35], as well as to distinguish different disease levels[36], showing promising discrimination to monitor rapidly, noninvasively, and cost-effectively. The chemical emissions are released to the surrounding gas phase from host plants, from which one can detect damage information from the pest-induced specific volatiles (i.e., volatile fingerprints) (Fig. 2). The sensor responses are consistent with the different VOC compositions which might be compared to the overall variation of a pool of reference gas samples[37].
Metal oxide semiconductor (MOS) gas sensors have been widely used in e-noses to distinguish VOCs due to their low manufacturing price and large response range[38]. For detecting target VOCs, metal oxide materials such as ZnO, SnO2, and TiO2 have been employed as a sensing layer. Wen et al.[39] developed a sweeping e-nose system for detection of citrus fruits infestation at early stages, containing a detection unit that is composed of MOS sensors and measurement & pattern recognition modules (Fig. 3). Thus, VOCs have been identified for differentiating citrus fruits infested with Bactrocera dorsalis, basing on measurements of the change of semiconductors conductivity in the presence of redox reactions on the sensitive material surface. Lampson et al.[40] developed a portable device to draw volatiles from pests or pest-damaged products over carbon black–polymer composite sensors and measure the change in resistance for each sensor. Furthermore, Biondi et al.[41] used a commercial e-nose equipped with a metal oxide sensor array to detect diseased potatoes infected with Ralstonia solanacearum and Clavibacter michiganensis subsp. sepedonicus, respectively achieving 81.3% and 57.4% classification of the samples in dynamic and static sampling procedures (active and passive gas-sampling). Additionally, the passive sampling allows effective discrimination in simulated conditions of bulk storage. Higher flow rates and appropriate sorbent materials may improve VOCs concentration effects to certain degrees for better discrimination[37].
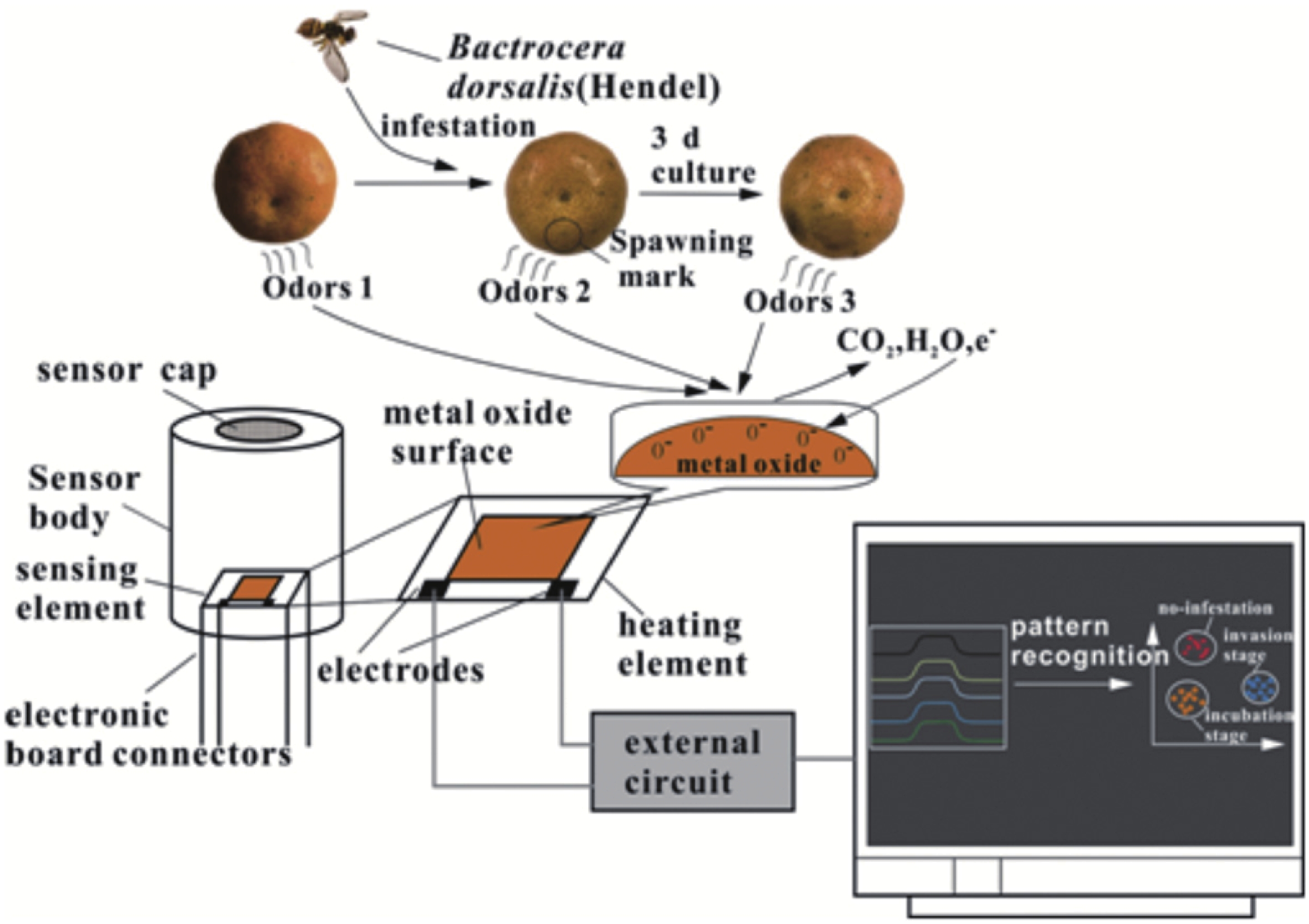
The nanomaterials-based biosensors have been widely-studied for sensing applications, particularly in electrochemical biosensing[42-44]. Carbon nanotubes (CNTs) are expected to have a mix of good electrical properties and VOC sensing ability, as they have exceptional physical and chemical properties[45]. The behavior of carbon nanostructure polymer composite sensor devices is based on the level of response, which is dependent on both the nature and concentration of the adsorbed molecular species. Greenshields et al.[46] report the chemical sensors based on carbon nanostructure-poly(vinyl alcohol) (PVA) composites to detect four fungi species in melons, revealing the sensors response to a stimulus (interaction with carbon nanostructure polymer composites) provided by the volatiles exhaled by fruits. The research team further developed a set of resistive sensors based on carbon nanostructures for fast detection and identification of two general of fungi (Rhizopus sp. and Aspergillus sp. section Nigri) on the strawberry fruits[47]. Moreover, Zhao et al.[48] functionalized the CNTs with carboxylic group to enhance the sensing properties, resulting that this sensor array was able to detect various VOCs with relatively high sensitivity. Furthermore, Verma et al.[49] demonstrated that “chemical nose” biosensors with gold nanoparticles can detect polymicrobial mixtures and identify bacteria with high accuracy. Besides, a promising approach has been proposed to exhibit high sensitivity for representative VOCs by conjugating a thiolated ligands on the molybdenum disulfide (MoS2) surface. Such surface modification may develop new ways to improve detection properties in the real-time VOC monitoring system, leading to more valuable applications for biological sensing[50]. Despite present biosensors are mostly proposed in environment safety and medical healthcare area, the fabrication of nanostructured biosensor has a potential for further applications on in-situ detection of agricultural monitoring.
In addition to detecting plant VOCs, the technology that capture pests volatile pheromone in sensitive manners would be attractive for detection before onset of pest infestation. Moitra et al.[51] developed the β-cyclodextrinylated MEMS devices for selective and sensitive detection of female sex pheromone of olive fruit pest, Bactocera oleae, and the detection limit of the devices has been achieved to a value as low as 0.297 ppq.
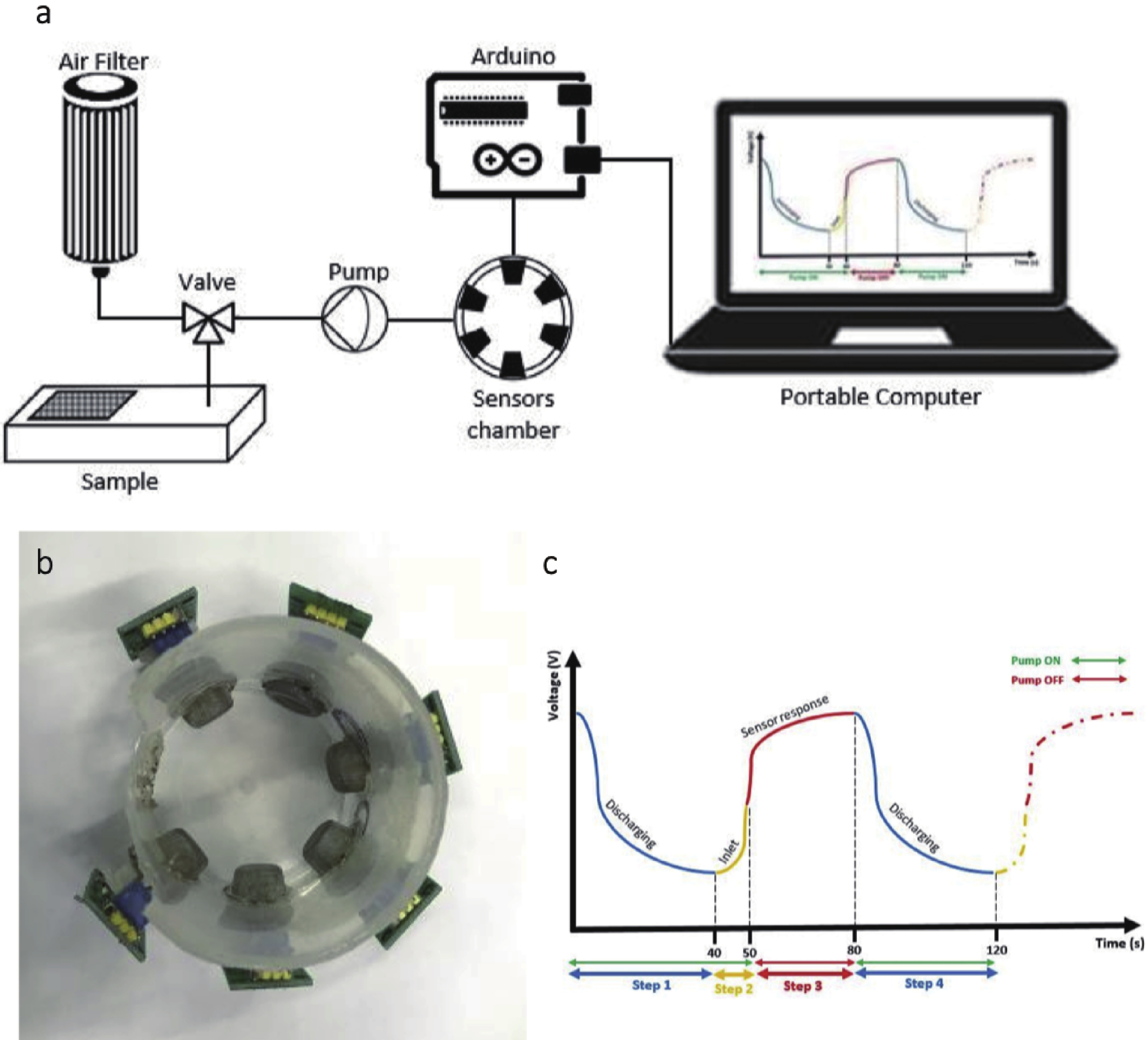
2.3 Wearable technology
Despite the rapid development of wearable biosensors that make use of stretchy and flexile electronics, their application potential to monitor plant health has not been fully developed[52]. Plant surfaces show far more diverse microscale characteristics than the human epidermis, which play a vital role in the exchange of substances and energy between plants and nature[53]. Recent progresses on flexible electronic technology in the field of agriculture enable monitoring on leaves or stems of plants to measure subtle changes[54, 55]. Wearable biosensors, developed to assess the status of plant health, have been exploited to measure the physiological and biochemical variations continuously by profiling relevant trait and microclimate parameters. The nanomaterials-based biosensors feature high flexibility and excellent mechanical strength, improving accessibility of miniaturized and portable devices in the fields of wearable bioelectronic devices, which might meet the demands for on-site monitoring and early detection[43, 56]. For example, flexible, stretchable and wearable carbon nanotube/graphite sensors were worn on the fruits of Solanum melongena and Cucurbita pepo, connecting with a readout circuit to make the real-time measurement of plant growth.
Developing chemical sensors that can monitor plant sap flow enables comprehensive monitoring of plant health and provides possibilities for early detection of abiotic and biotic stresses. Recently, a flexible electronic sensor (Figs. 4(a) and 4(b)) was reported, that can harmlessly cohabitate with the plant and continuously monitor sap flow of plants in the complex environment (e.g., agricultural farm field or greenhouse)[53]. The measurements of sap flow rates provide a real-time tracking method to study plant behaviors by analyzing plant health, water consumption, and nutrient distribution. The flexible and shape-morphing sensor systems were proposed for the nondestructive and long-term integration with plant organs[57]. For instance, the leaf temperature sensor is based on the porous substrate to continuously monitor microenvironment temperature without causing any physical damage and showing excellent biocompatibility. The flexible humidity sensors are able to assess the plant transpiration processes that exchange water molecules with the ambient basing on the opening and closing of stomata[58]. Lan et al.[59] used graphene oxide (GO) as the humidity-sensitive material to produce a flexible capacitive-type GO-based humidity sensor attaching to the lower surface of a leaf (Fig. 4(c)). Besides, a tiny graphene sensor that taped to plants was developed to monitor plant transpiration process and water status[60]. It is worth noted that flexible devices fabricated with graphene and its derivatives have shown great potential for non-destructive monitoring of plant health.
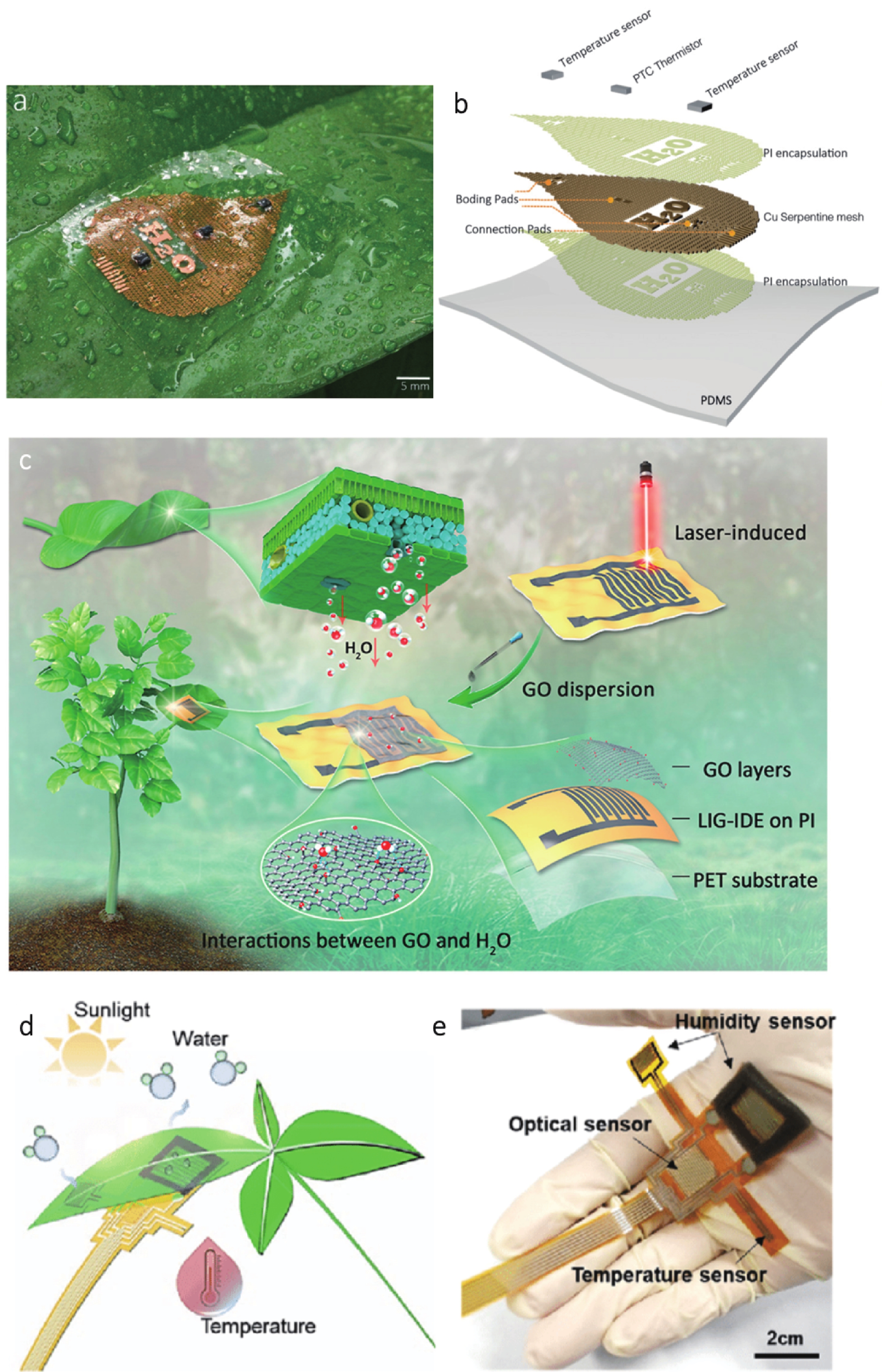
Wearable plant sensors hold great promise for plant stresses detection, which could monitor variations on tissue temperature, transpiration rate, or aperture of stoma. However, plants’ responses to pests and diseases are far more complicated and hard to diagnose specifically through those limited physiological activities. Therefore, multimodal sensing systems with high sensitivity and selectivity need to be further developed in a complicated way[11, 61]. Lu et al.[62] proposed an integrated multimodal flexible sensor system using ZnIn2S4 (ZIS) nanosheets to monitor the plant status by investigate the factors pertaining to plant health (Fig. 4(d)). The system consists of a temperature sensor, a humidity sensor for ambient moisture measurement, a leaf-surface humidity sensor for plant monitoring, and an optical sensor (Fig. 4(e)). In particular, the humidity sensors are applied to monitor the ambient humidity and plant transpiration. Khan et al.[63] reported an ultralightweight flexible sensory platform based on bare die complementary metal oxide semiconductor (CMOS) chips, holding a light, temperature, and humidity sensor on a flexible polymer substrate. The platform that placed on the plant leaf is applied to monitor microclimate conditions surrounding a plant for accurate growth monitoring. Using flexible and biocompatible materials coupled with a smart compact design, Nassar et al.[64] developed compliant plant wearables integrating temperature, humidity and strain sensors, which can be intimately deployed on the soft surface of any plant to remotely and continuously evaluate optimal growth settings. A flexible electronic device, based on the integration of single-walled carbon nanotube (SWCNT) channels and graphitic electrodes, was mounted on the leaf surface for sensing organic vapor in air[65]. In addition, a wearable platform that could measure gas emissions was developed for real-time monitoring and early detection of plant diseases. Li et al.[66] reported a leaf-attachable chemiresistive sensor array for noninvasive diagnosis of late blight caused by Phytophthora infestans. The sensor was designed by integrating a graphene-based sensing material and flexible silver nanowire (AgNW) electrode on a stretchable kirigami-based substrate. The sensing system enables early detection (within 4 days of inoculation) of P. infestans infection with high sensitivity, and monitoring of abiotic stresses such as mechanical injuries. Moreover, recent studies have proposed that magnesium-ion batteries (MIB) could be used as a flexible integrated unit to power future self-powered systems[67]. As a promising technology, wearable electronics are capable of continuous monitoring to track the status of plant health in real-time, and are expected to apply for monitoring and detection of crop pests and diseases.
3. Conclusions, challenges and future perspectives
Overall, each of the reviewed technologies shows distinct advantages over traditional monitor methods toward continuous monitoring and automation, not only providing a path to detect pests or diseases at a much earlier stage, but also generating precise, high-resolution and multidimensional data. Monitoring techniques based on morphology, bio-markers regarding plant physiology and biochemistry (e.g., VOCs, metabolite), and the microclimate relevant to bioprocesses allow early detection of pests and diseases. The advanced sensing technologies provide unprecedented opportunities for more specific analyses to generate novel insights into interactions among individual organisms and environments, and thus offer a sustainable solution for effective monitoring for biosecurity.
Integration among technologies must be involved to improve plant health management and enhance crop production efficiency. It is indispensable to require interdisciplinary collaborations among plant science, electronic engineering and data science, which are likely to be future trends. Such integration is essential for efficient cocreation and advanced solutions to ensure the practical application being fit-for-purpose. These technologies include semiconductor engineering, wireless sensor network, data processing and management, as well as biology. The cooperation of agriculture experts would investigate the biometric signature of particular species, further to detect and identify pests and to diagnose the causal agents of diseases. Biosensor-based monitoring generate a large set of multidimensional data that can be transformed to biological information. For example, image-based monitoring approaches along with deep learning algorithms are developed for trapped insect detection to recognize and count the number of individuals[68]. Moreover, data collection can be automated with sensor technologies and robotics, widening possibilities for autonomous monitoring and providing indications at scales across the microscopic to landscape levels[69]. Unmanned aerial vehicles (UAVs) have been increasingly adopted for crop monitoring and disease detection due to the accessibility of high-solution image sensing and easy-to-use for in-field operation[70]. An approach to combine UAVs with sticky traps was proposed for automated detection of Drosophila suzukii, highlighting the detection potential of UAV imagery to monitor insects[71]. Besides, ground-based platforms for automated monitoring offer greater flexibility that provide fine-scaled data and minimize the disturbances to crops as well (legged robots) [72-74]. The potential of integrated technologies requires further exploration to achieve overall aims, and thus could provide unprecedented opportunities for applications of agriculture monitoring.
The advancements of the biosensing methodology have opened up new avenues for monitoring of pests and diseases, however, practical applications for long-term monitoring, accurate detection, and identification are still in the developmental phases. One of the challenges is processing multidimensional data into reliable measurements, which can be further translated into biological information for status assessment and decision making. As the alterations in biological characteristics captured in field may occur under biotic and abiotic stress combinations (e.g., mixed infections), to specifically discern the effects caused due to individual stressors remains a challenging task. It is necessary to build biosensor systems with high sensitivity and selectivity to meet demands for in-field monitoring. At the same time, the stability should be considered due to the variability of environmental factors. The high investment costs impede steps toward the continuous monitoring and automation to some extent, however, advancements in power sources and scheduling of operations will enable the long-term field deployment in a relatively cost- and power-effective way[75, 76]. Besides, novel hardware and software approaches for data storage and wireless data transmission are being explored, further upscaling agriculture monitoring and allowing real-time data capture[77]. Technological innovations are creating opportunities to allow effective monitoring and detection for precise agriculture and biosecurity. It will become a trend to use advanced biosensors strategically to meet specific needs for accurate, real-time and automated monitoring.
Acknowledgements
The work is supported by National Key Research and Development Program of China (Grant No. 2022YFC2602100) and Chinese Academy of Inspection and Quarantine (2022JK38).